The up to date Mehtyl-IT version is part of EpiMethyl Analytic Technology, which is currently included into EpiCrops Technologies.
The analysis of every new methylation data set with Methyl-IT leads to discovery of new knowledge, leading to almost a continuous increment of the value of EpiMethyl Analytic Technology.
Methyl-IT applies methods described in the US patent US20170218463A1
Projects
Uncovering human, animal, and plant DNA methylation codes with the application of machine learning algorithms, multivariate statistics and mathematical modeling
Methyl-IT Development
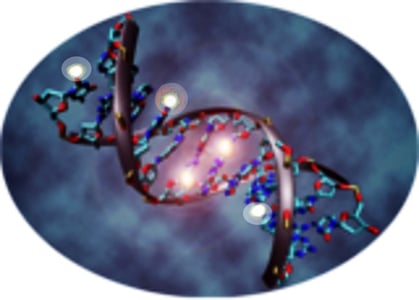
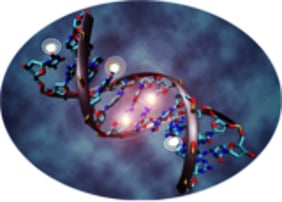
Understanding The Methylation Code
Analysis of genome-wide methylation patterns to understand their role in gene regulation.
Identification of differentially methylated regions associated with diseases.
Characterization of epigenetic modifications during development and aging.
Integration of Methyl-IT with other omics data for comprehensive analysis.
Collaborative projects with researchers to apply Methyl-IT in diverse biological studies.
Development of user-friendly interfaces and tools for easy accessibility of Methyl-IT.
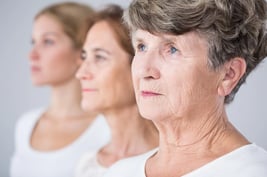
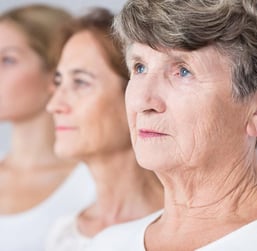
To Date Applications
Methyl-IT basic biomedical application permits the discrimination of healthy individual (for given disease) from patients. In particular, application for cancer diagnostic is almost trivial, since the methylation signal induced by cancer cells is very strong.
The analysis of leukemia patients was reported in reference (5). Applications to the analysis of plants, animals, and humans are focused on pathophysiological processes that require for very sensitive analytical approaches.
Human brain
Patients with neurodegenerative disorders
Placenta tissue from patients with Autism Spectrum Disorder
Patients with Type II Diabetes
Patients with different types of cancer
For all the mentioned data sets the identification the discrimination of healthy individual (for a given disease) from patients is straightforward with MethylIT. So, the further development of our technology is focussed on unveiling the gene networks and sub-networks of hubs that play a major rule on the disease progressing. That is, our technology departs from the classic reductionist biomarker identification (typically followed by the biofarm companies in drug designing) which oversimplify human defense molecular mechanisms and homoestasis.
Currently, we have analyzed the following human methylation data sets:
Application in plant epigenomics can be read in our published reports
Please see
An application in Coral
Network-associated responses resulted in the identification of hub genes and clusters associated to the change in phenotype: inter-partner recognition and phagocytosis, soft tissue growth and biomineralization. Furthermore, we identified hub genes putatively involved in animal photoreception–phototransduction. These findings fundamentally advance our understanding of how reef-building corals repattern the methylome and adjust a phenotype, revealing an important role of light sensing by the coral animal to optimize photosynthetic performance of the symbionts. (7)
References
Sanchez R, Mackenzie SA. On the thermodynamics of DNA methylation process. Sci Rep. 2023;13: 8914. doi:10.1038/s41598-023-35166-9.
Sanchez, R.; Yang, X.; Maher, T.; Mackenzie, S.A. Discrimination of DNA Methylation Signal from Background Variation for Clinical Diagnostics. Int. J. Mol. Sci. 2019, 20, 5343.
Sanchez R, Mackenzie SA: Genome-Wide Discriminatory Information Patterns of Cytosine DNA Methylation. Int. J. Mol. Sci. 2016, 17(6), 938.
Sanchez R, Mackenzie SA: Information Thermodynamics of Cytosine DNA Methylation. Plos One 2016, 11.
Sanchez R, Mackenzie SA: Integrative Network Analysis of Differentially Methylated and Expressed Genes for Biomarker Identification in Leukemia. Sci Rep 2020, 10(1), 2123.
Yang, X, Sanchez, R et al.: Segregation of an MSH1 RNAi transgene produces heritable non-genetic memory in association with methylome reprogramming. Nature Communications 2020, 11(1), 2214
Gomez-Campo, K., Sanchez, R., Martinez-Rugerio, I., Yang, X., Maher, T., Osborne, C. C., Enriquez, S., Baums, I. B., Mackenzie, S. A., & Iglesias-Prieto, R. (2023). Phenotypic plasticity for improved light harvesting, in tandem with methylome repatterning in reef-building corals. Molecular Ecology, 00, e17246. https://doi.org/10.1111/mec.17246